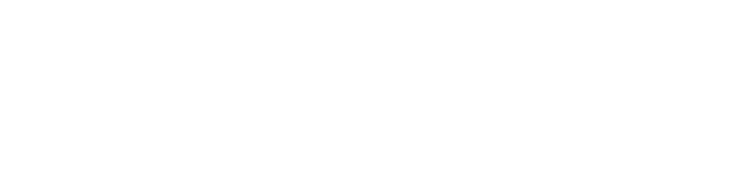

Should We Be Worried About the Black Box Problem
This article discusses the black box problem in Artificial Intelligence (AI), where the process used by AI is unknown, and how it can lead to biased decision-making. The solution to this problem lies in Explainable AI, where the algorithm itself comes with an explanation for its results as a part of its design. The document also highlights the importance of keeping humans in the loop of developing AI to minimize the harmful effect that biases in AI can have.
In 2019, Apple launched its Apple Card, a credit card featured on Apple users’ phone. The brand says users can apply and know if they’re approved in just minutes, without impacting their credit score. The credit card offers features like facial recognition and fingerprint scan to enhance safety and privacy. But despite all these benefits, after its launch the card became quite controversial. Even Apple co-founder Steve Wozniak weighed in on the discussion, revealing on Twitter that his wife got a credit score ten times lower than he got, even though they share all their assets. He also twittered that even though he contacted the company, he got nowhere.
This is a telling example of the so-called black box problem. We don’t know the process that is used by Artificial Intelligence, only the output. Therefore, the name ‘’black box’’. We also don’t know exactly how our brains work and ultimately that’s what deep learning is trying to replicate, using neural networks.
Why it is a problem
So why is it a problem? Artificial Intelligence offers many solutions and benefits, enhancing effectivity and lowering costs for businesses. But with AI, and in particular deep learning systems being used more and more to replace normally human-performed decisions, its crucial to create systems that offer some type of insight and explanation for the way it operates. Just like in the case of the Apple Card, a lot of banks use AI to make decisions involving credit scores and loans. These are decisions which have the potential of impacting peoples’ lives greatly, making it important to know why and how these systems give the output they do. One of the factors contributing to this problem is that when AI is trained with bias, the bias stays present in the system and influences that same system’s output. Deep learning networks tend to be extremely difficult to understand and explain, and sometimes this leads to the dilemma of choosing a more complex system that works better or choosing a less accurate system, but with a higher understandability. So applicating this to the case where a client’s loan is rejected, the algorithm would have to explain or offer some type of insight why it came to that conclusion. Black box AI makes it difficult for programmers to control their systems and detect bias, because they don’t know which factors in information are weighed to create output.
Solving the problem
The solution to this problem lies within Explainable AI. First, there’s a key distinction to be made. Explainable AI doesn’t necessarily mean that the systems' output should be more understandable for humans, it means that the algorithm itself comes with an explanation for its results as a part of its design. According to the director of the New York software company SparkBeyond, Brian D’Alessandro, programmers should take in account what is appropriate for the human cognitive scale. "Most people can fully consume a rule that has five or six different factors in it. Once you get beyond that, the complexity starts to get overwhelming for people", he stated. There is a movement towards Explainable AI. For instance, New York legislation now mandates a so called ‘bias audit’ for employers to use AI systems to recruit new employees. This means that a group of independent reviewers will run the program and see what impact it has on the non-variable components such as ethnicity or gender, reporting its results back to the employer. This way companies can get feedback on the impact of their systems, and understand the way it can potentially affect users.
So, by keeping humans in the loop of developing AI to make decisions also made by humans (for example hiring employees or granting a loan), developers can keep a closer eye on the effect their program has on users, minimizing the harmful effect that biases in AI can have. Besides that, explainable AI could be just the right solution to combat the black box problem. Programmers should take into account what humans are able to understand when designing their technology. That way the complexity and quantity of factors of AI output won’t overwhelm people.
Have we sparked your interest to learn more about all the possibilities in the world of AI? Contact us via our Baise website and let us know what you're looking for. We offer our personal advice and expertise on AI for your company. Our aim is to connect brains with business by providing you with talented and ambitious students looking to gain real experience in the field.
More Articles
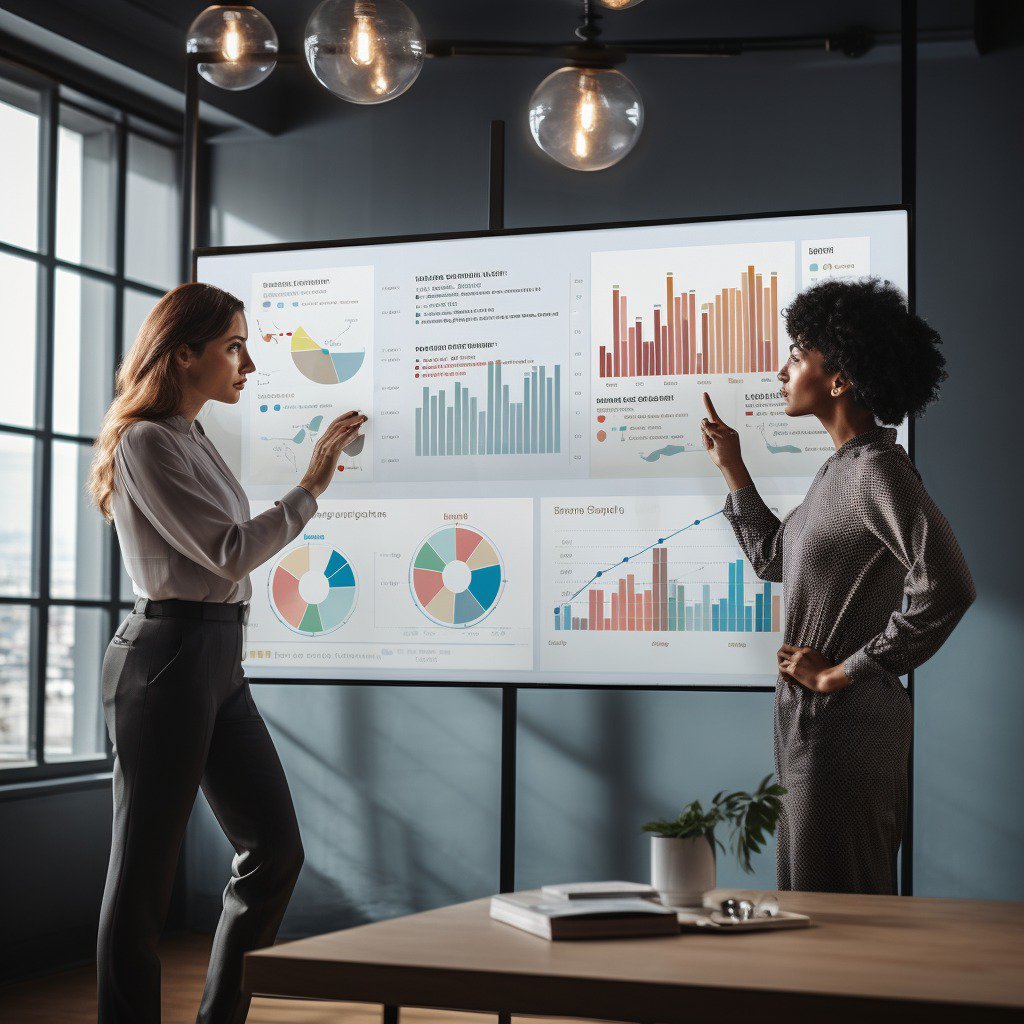